Revolutionizing healthcare: the role of artificial intelligence in clinical practice
Shuroug A. Alowais, Sahar S. Alghamdi, Nada Alsuhebany
Introduction
Healthcare systems are complex and challenging for all stakeholders, but artificial intelligence (AI) has transformed various fields, including healthcare, with the potential to improve patient care and quality of life. Rapid AI advancements can revolutionize healthcare by integrating it into clinical practice. Reporting AI’s role in clinical practice is crucial for successful implementation by equipping healthcare providers with essential knowledge and tools.
Research Significance
This review article provides a comprehensive and up-to-date overview of the current state of AI in clinical practice, including its potential applications in disease diagnosis, treatment recommendations, and patient engagement. It also discusses the associated challenges, covering ethical and legal considerations and the need for human expertise. By doing so, it enhances understanding of AI’s significance in healthcare and supports healthcare organizations in effectively adopting AI technologies.
Materials and Methods
The current investigation analyzed the use of AI in the healthcare system with a comprehensive review of relevant indexed literature, such as PubMed/Medline, Scopus, and EMBASE, with no time constraints but limited to articles published in English. The focused question explores the impact of applying AI in healthcare settings and the potential outcomes of this application.
Results
Integrating AI into healthcare holds excellent potential for improving disease diagnosis, treatment selection, and clinical laboratory testing. AI tools can leverage large datasets and identify patterns to surpass human performance in several healthcare aspects. AI offers increased accuracy, reduced costs, and time savings while minimizing human errors. It can revolutionize personalized medicine, optimize medication dosages, enhance population health management, establish guidelines, provide virtual health assistants, support mental health care, improve patient education, and influence patient-physician trust.
Conclusion
AI can be used to diagnose diseases, develop personalized treatment plans, and assist clinicians with decision-making. Rather than simply automating tasks, AI is about developing technologies that can enhance patient care across healthcare settings. However, challenges related to data privacy, bias, and the need for human expertise must be addressed for the responsible and effective implementation of AI in healthcare.
Methodology for illness detection by data analysis techniques
Vira Liubchenko*, Nataliia Komleva, Svitlana Zinovatna, Jim Briggs
The research aims to develop information technology for identifying problematic health conditions by analyzing measurement data. The literature review highlights various approaches to medical diagnostics, including statistical and machine-learning models that predict the risk of adverse outcomes based on patient data. Developed information technology focuses on data classification and sufficiency, ensuring objective and relevant data is collected. The technology involves expert-defined rules for analysis, aiding in generating patient diagnosis candidates. The proposed information system comprises four components: data source, data storage, diagnosis module, and data sink. A comprehensive data storage structure is designed to store and manage data related to diagnoses and parameters efficiently. The rule set generation block prototype includes obtaining parameters and transforming algorithms into programming functions. A case study focuses on a diagnostic tool for assessing PTSD using an internationally recognized questionnaire. Telegram bot is selected as the data source due to its anonymity, flexibility, and automated data collection capabilities. The database structure is designed to accommodate questionnaire modifications and continue data collection. The implemented analytical system effectively categorizes individuals’ states based on their responses. Overall, the research demonstrates the potential of information technology and the proposed information system to provide effective and user-friendly health diagnostics, aiding in timely medical interventions and improving population well-being.
AI for the detection of diseases – Image analysis
In recent years, artificial intelligence has played a prominent role in the area of health.
Its booming use has to fulfil various technical, legal, policy and budgetary criteria.
1. Technical aspects
Artificial intelligence (AI) is currently reshaping the world, especially in the area of health. Indeed, it is easily able to detect various types of diseases such as cancers and tumours, even at an early stage of their development.
Models based on AI are being developed based on a very large quantity of healthcare training data, in the form of medical images such as X-rays, CAT scans, MRIs and all other types of imaging results.
Examples of AI detection capacities in medical imaging
- Detection of neurological anomalies
- Detection of common cancers (breast, lung cancer, etc.)
- Diagnosis of kidney and liver infections
- Detection of brain tumours with a high level of accuracy
- AI machine learning for the analysis of dental images
- Detection of bone fractures and musculoskeletal lesions
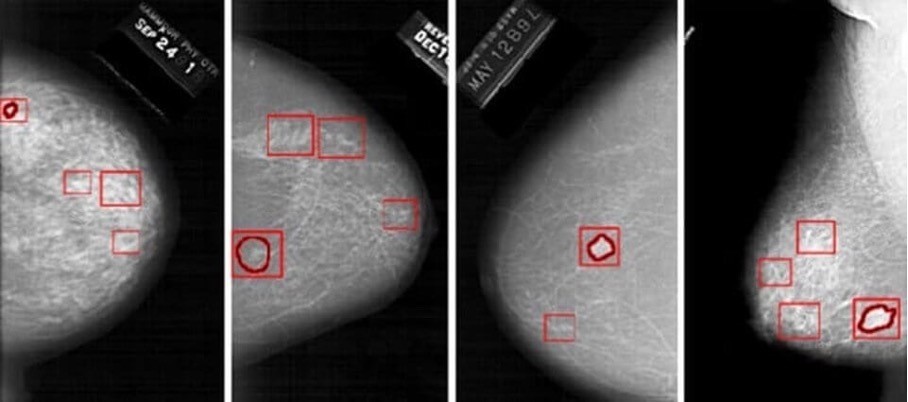
The risk indicated by the model can lead the doctor to detect traces of cancer at a very advanced stage that would not have been seen with fatigue or overwork or poor-quality images.
It must be noted that the doctor always makes the final decision concerning the patient’s diagnosis. AI provides guidance but never issues an opinion concerning the diagnosis or the measures to be taken.
This legal aspect of AI in healthcare is addressed below
2. Legal aspects
Faced with the fast pace of technological progress in the area of AI and in light of the associated risks in terms of safety and fundamental rights, the European Commission has proposed a much-anticipated legal framework in the form of a draft regulation associated with a coordinated action plan (the Artificial Intelligence Act). The text was submitted in April 2021 but is still under discussion to harmonise the various countries’ points of view. While no date has been set for the moment, the text should be adopted in 2023.
The principles of quality, integrity, safety, robustness, resilience, supervision, explainability, interpretability, transparency, reliability, traceability, human oversight and auditability, which were previously identified in various European texts and have been implemented by developers and publishers by means of more or less efficient governance systems, are addressed and translated into specific obligations for providers starting from the design of an AI system and then throughout its life cycle.
This set of requirements reinforces, in the form of legislation, best practices applicable to the development and monitoring of high-risk AI systems, including the obligations of providers and professional users, and defines conditions of governance as well as penalties for infringing.
In the healthcare sector, given the challenges associated with the use of AI systems as decision-making and diagnostic tools, the relevance and accuracy of AI data shall be guaranteed, requiring that these systems be placed on the market in accordance with the Regulations on medical devices (Regulation (EU) 2017/745) and data protection (Regulation (EU) 2016/679). The validity of a high-risk AI system shall be assessed in terms of its compliance from the design stage and then throughout its life cycle through the establishment, in particular, of an iterative risk management system.
The technical documentation of a high-risk AI system shall be drawn up before that system is placed on the market or put into service and shall be kept up to date. It shall demonstrate that the high-risk AI system complies with the requirements of the Artificial Intelligence Act and shall thus provide notified bodies and competent authorities with all the information necessary to place the system on the market or put it into service.
Machine learning AI systems shall be developed based on high-quality training, validation and testing datasets subject to appropriate data management and governance practices. The Artificial Intelligence Act notes that to the extent that it is strictly necessary for the purposes of ensuring bias monitoring, detection and correction in relation to high-risk AI systems, the providers of such systems may process special categories of personal data, including health data, subject to appropriate safeguards such as pseudonymisation or encryption where anonymisation may significantly affect the purpose pursued (example of clinical investigations). In France, it can still be complicated to access the datasets of
manufacturers with a view to developing AI systems. Similarly, for anonymised datasets, the legal conditions applying to anonymisation need to be specified, i.e. the qualifications of the processors, the applicable legal bases and the conditions of compliance, and the rights and freedoms of the persons concerned.
3. Policy and budgetary aspects
The public authorities have clearly understood the role played by digital technology in the area of health.
They have started developing a 5P (personalised, preventive, predictive, participatory and precision) approach to medicine to benefit citizens, patients and the healthcare system. This approach is based for example on connected objects, service platforms, AI, digital medical devices, digital twins, trial simulators, robotics, and many other devices.
On 29 June 2022, the President of the French Republic presented the over €7.5 billion “Health Innovation” 2030 plan at the Strategic Council of Health Industries (CSIS). The aim is to make France one of Europe’s leading innovative nations in the area of health.
Furthermore, the €650 million “Santé Numérique” acceleration strategy under the “Investissements d’avenir” programme is promoting the development, validation and testing of digital tools for this 5P approach to medicine.
To learn more and read about these financing arrangements, visit the following page: Investissements d’avenir: launch of the “Santé Numérique” acceleration strategy | enseignementsup-recherche.gouv.fr
CONCLUSION:
AI in health is a growing practice that is automating recurring tasks for healthcare professionals so they may have more time to focus on activities that have real added value for patients.
AI and the digitisation of healthcare are also tools that are being used to combat the lack of doctors in certain regions and the decrease in the number of practising physicians.
However, let us make no mistake: the aim is not to replace doctors with machines; rather, it is to organise virtuous interactions between human expertise and AI systems.
Efor, the European leader in life sciences consulting, assists you with the technical and regulatory aspects of the development process for your AI systems.
Our experts will be pleased to provide you with support through the various stages of your product’s development, from its design to its maintenance on the market.
Contact them directly by writing to onedt@efor-group.fr
Better diagnosing diseases with the help of AI
Researchers improve the trustworthiness of medical imaging diagnoses with innovative three-stage system powered by AI
An interdisciplinary team of researchers at the University of Waterloo has developed a more trustworthy method to diagnose diseases such as COVID-19, pneumonia, and melanoma using artificial intelligence (AI) tools.
Waterloo engineering professor Alexander Wong teamed up with other researchers from the university, McGill University, and the National Research Council of Canada to develop a new method to support doctors by enhancing computer-aided diagnosis through deep learning.
The researchers created a system they’ve dubbed Trustworthy Deep Learning Framework for Medical Image Analysis (TRUDLMIA), which leverages the power of supervised and self-supervised AI learning that aims to pave the way for advancements in high-performing and trustworthy healthcare models.
“Models employing the TRUDLMIA approach outperform existing ones in various tasks, excelling in COVID-19, pneumonia, and melanoma diagnosis, surpassing models specifically designed for those tasks in terms of both performance and trust,” said project lead Dr. Wong, the Canada Research Chair in Medical Imaging and Artificial Intelligence. “The proposed system is undergoing further development to address future pandemics and syndromes, including long-term effects associated with COVID-19.”
Medical imaging and deep learning can improve medical AI by assisting with diagnosis, prediction and prognosis. To date, a key issue in using deep learning for medical image analysis has been the presence of data bias, low trust and accountability, and challenges in interpreting existing data.
TRUDLMIA’s innovation works in three stages to train the AI system. First, it learns from a very large set of labelled general data in a pre-training process, after which it learns from both general data and domain-specific data (e.g., medical data) without labels in a self-supervised learning process. Finally, it fine-tunes the AI with task-specific labelled data (e.g., clinical diagnosis data) with a special objective that focuses on handling data imbalances and biases and improving AI trustworthiness.
“TRUDLMIA, developed collaboratively with medical doctors, is poised to transform medical image analysis,” said collaborator Dr. Pengcheng Xi, Senior Research Officer at the National Research Council of Canada. “With a focus on improving diagnostic accuracy, boosting trust, and adapting across medical specializations, TRUDLMIA aspires to be an ally for doctors, to provide timely interventions for patients, and to support caregivers through preventive care.”
More information about this work can be found in the research paper, “Towards Building a Trustworthy Deep Learning Framework for Medical Image Analysis,” published recently in the journal Sensors.
Reference:
https://uwaterloo.ca/news/media/better-diagnosing-diseases-help-ai
European validation of an image-derived AI-based short-term risk model for individualized breast cancer screening—a nested case-control study
Mikael Eriksson, Marta Román, Axel Gräwingholt
Summary
Background
Image-derived artificial intelligence (AI)-based risk models for breast cancer have shown high discriminatory performances compared with clinical risk models based on family history and lifestyle factors. However, little is known about their generalizability across European screening settings. We therefore investigated the discriminatory performances of an AI-based risk model in European screening settings.
Methods
Using four European screening populations in three countries (Italy, Spain, Germany) screened between 2009 and 2020 for women aged 45–69, we performed a nested case-control study to assess the predictive performance of an AI-based risk model. In total, 739 women with incident breast cancers were included together with 7812 controls matched on year of study-entry. Mammographic features (density, microcalcifications, masses, left-right breast asymmetries of these features) were extracted using AI from negative digital mammograms at study-entry. Two-year absolute risks of breast cancer were predicted and assessed after two years of follow-up. Adjusted risk stratification performance metrics were reported per clinical guidelines.
Findings
The overall adjusted Area Under the receiver operating characteristic Curve (aAUC) of the AI risk model was 0.72 (95% CI 0.70–0.75) for breast cancers developed in four screening populations. In the 6.2% [529/8551] of women at high risk using the National Institute of Health and Care Excellence (NICE) guidelines thresholds, cancers were more likely diagnosed after 2 years follow-up, risk-ratio (RR) 6.7 (95% CI 5.6–8.0), compared with the 69% [5907/8551] of women classified at general risk by the model. Similar risk-ratios were observed across levels of mammographic density.
Interpretation
The AI risk model showed generalizable discriminatory performances across European populations and, predicted ∼30% of clinically relevant stage 2 and higher breast cancers in ∼6% of high-risk women who were sent home with a negative mammogram. Similar results were seen in women with fatty and dense breasts.
Funding
Swedish Research Council.
Artificial intelligence in disease diagnostics: A critical review and classification on the current state of research guiding future direction
Stefan Stieglitz
The diagnosis of diseases is decisive for planning proper treatment and ensuring the well-being of patients. Human error hinders accurate diagnostics, as interpreting medical information is a complex and cognitively challenging task. The application of artificial intelligence (AI) can improve the level of diagnostic accuracy and efficiency. While the current literature has examined various approaches to diagnosing various diseases, an overview of fields in which AI has been applied, including their performance aiming to identify emergent digitalized healthcare services, has not yet been adequately realized in extant research. By conducting a critical review, we portray the AI landscape in diagnostics and provide a snapshot to guide future research. This paper extends academia by proposing a research agenda. Practitioners understand the extent to which AI improves diagnostics and how healthcare benefits from it. However, several issues need to be addressed before successful application of AI in disease diagnostics can be achieved.
Artificial Intelligence for Hospital Health Care: Application Cases and Answers to Challenges in European Hospitals
Matthias Klumpp
The development and implementation of artificial intelligence (AI) applications in health care contexts is a concurrent research and management question. Especially for hospitals, the expectations regarding improved efficiency and effectiveness by the introduction of novel AI applications are huge. However, experiences with real-life AI use cases are still scarce. As a first step towards structuring and comparing such experiences, this paper is presenting a comparative approach from nine European hospitals and eleven different use cases with possible application areas and benefits of hospital AI technologies. This is structured as a current review and opinion article from a diverse range of researchers and health care professionals. This contributes to important improvement options also for pandemic crises challenges, e.g., the current COVID-19 situation. The expected advantages as well as challenges regarding data protection, privacy, or human acceptance are reported. Altogether, the diversity of application cases is a core characteristic of AI applications in hospitals, and this requires a specific approach for successful implementation in the health care sector. This can include specialized solutions for hospitals regarding human–computer interaction, data management, and communication in AI implementation projects.
Reference:
A comparison of deep learning performance against health-care professionals in detecting diseases from medical imaging: a systematic review and meta-analysis
Xiaoxuan Liu, Livia Faes
Summary
Background
Deep learning offers considerable promise for medical diagnostics. We aimed to evaluate the diagnostic accuracy of deep learning algorithms versus health-care professionals in classifying diseases using medical imaging.
Methods
In this systematic review and meta-analysis, we searched Ovid-MEDLINE, Embase, Science Citation Index, and Conference Proceedings Citation Index for studies published from Jan 1, 2012, to June 6, 2019. Studies comparing the diagnostic performance of deep learning models and health-care professionals based on medical imaging, for any disease, were included. We excluded studies that used medical waveform data graphics material or investigated the accuracy of image segmentation rather than disease classification. We extracted binary diagnostic accuracy data and constructed contingency tables to derive the outcomes of interest: sensitivity and specificity. Studies undertaking an out-of-sample external validation were included in a meta-analysis, using a unified hierarchical model. This study is registered with PROSPERO, CRD42018091176.
Findings
Our search identified 31 587 studies, of which 82 (describing 147 patient cohorts) were included. 69 studies provided enough data to construct contingency tables, enabling calculation of test accuracy, with sensitivity ranging from 9·7% to 100·0% (mean 79·1%, SD 0·2) and specificity ranging from 38·9% to 100·0% (mean 88·3%, SD 0·1). An out-of-sample external validation was done in 25 studies, of which 14 made the comparison between deep learning models and health-care professionals in the same sample. Comparison of the performance between health-care professionals in these 14 studies, when restricting the analysis to the contingency table for each study reporting the highest accuracy, found a pooled sensitivity of 87·0% (95% CI 83·0–90·2) for deep learning models and 86·4% (79·9–91·0) for health-care professionals, and a pooled specificity of 92·5% (95% CI 85·1–96·4) for deep learning models and 90·5% (80·6–95·7) for health-care professionals.
Interpretation
Our review found the diagnostic performance of deep learning models to be equivalent to that of health-care professionals. However, a major finding of the review is that few studies presented externally validated results or compared the performance of deep learning models and health-care professionals using the same sample. Additionally, poor reporting is prevalent in deep learning studies, which limits reliable interpretation of the reported diagnostic accuracy. New reporting standards that address specific challenges of deep learning could improve future studies, enabling greater confidence in the results of future evaluations of this promising technology.
Diagnosing disease with AI could be the new norm in personalized medicine
A new AI diagnostic tool uses microbiome data and lifestyle factors to predict risk of multiple diseases, ushering in a new era of personalized healthcare.
Our bodies are brimming with trillions of microorganisms, including bacteria, fungi, parasites, and viruses. Collectively, these living entities make up our microbiome, which is shaped by our DNA, external environment, and diet.
The microbiome’s composition is unique to each individual and not only gives information about our current health status — even our emotional well-being — but also how well we age and the likelihood of developing chronic disease later in life.
Using artificial intelligence (AI), scientists have been able to correlate the composition of an individual’s gut with certain diseases, taking personalized medicine to the next level.
For example, last year, researchers introduced a microbiome-based predictive model that could accurately and non-invasively predict type 2 diabetes and inflammatory bowel disease (IBD), two ubiquitous non-communicable diseases. But this model did not take the possibility of comorbidities into account, limiting its practicality.
With the global shift toward population aging, a growing number of people are living with more than one chronic disease, a scenario often overlooked by healthcare practitioners.
To confront this reality, tools that facilitate the detection of multiple diseases simultaneously are needed, and a new AI-powered tool called Meta-Spec could do just that.
A personalized tool to assess health status
Meta-Spec, an AI-based diagnostic tool developed by researchers in China and the United States, considers multiple factors that might contribute to disease rather than relying solely on microbiome data. The result is a more nuanced, comprehensive approach to disease detection and prediction than what other models can currently offer.
Meta-Spec incorporates easily collected physical and lifestyle data such as diet, body mass index, and age. These details are part of a host’s “phenotype” — the collection of observable traits that stem from that person’s genes and their environment.
“By harnessing the power of deep learning and integrating it with microbiome data, Meta-Spec offers a glimpse into a future where healthcare is more personalized, more accurate, and ultimately, more effective,” stated Xiaoquan Su, a bioinformatics professor at Qingdao University’s College of Computer Science and Technology and one of Meta-Spec’s developers.
This multi-faceted approach significantly improves the model’s disease-screening accuracy to the point of being able to simultaneously detect multiple diseases, if present.
“In the past, the focus has largely been on detecting individual diseases, often overlooking the complex interplay of various factors that influence our health,” said Shunyao Wu, a computer science professor at Qingdao University and member of Meta-Spec’s development team.
Training Meta-Spec to detect diseases
Meta-Spec classifies diseases using multitask deep learning, a machine-learning technique that uses artificial neural networks to recognize patterns. Through several different “layers”, the model learns these patterns in a given dataset by merging different microbial features with questionnaire-based host data (“metadata”).
This can range from how often the host has vivid dreams to their bowel movement quality. From this data, the model learns to associate a distinct microbiome pattern with a particular disease, and each disease’s probability is calculated.
To train and validate their model, the researchers used data from several open-source platforms that collect human microbiome specimens from volunteers, including the American Gut Project and the Guangdong Gut Microbiome Project.
In the first dataset, each patient had been diagnosed with one or more diseases, including autoimmune disease, lung disease, thyroid disorder, cancer, IBD, cardiovascular disease, and autism spectrum disorder. Patients in the second dataset had metabolic syndrome, gastritis, type 2 diabetes, and/or gout.
The keys to Meta-Spec’s accuracy
For each dataset, Meta-Spec predicted the diagnosed disease(s) more accurately than traditional machine-learning methods. When only microbiome data was factored in, both Meta-Spec and models based on traditional methods performed much worse, demonstrating that the additional information about a person’s lifestyle — including seemingly unrelated details such as how often they floss their teeth — greatly improved the model’s predictive performance.
To further test their model’s capabilities, the researchers divided the two datasets into a single-disease group and a comorbidity group with one or more additional diseases. In this case, the model also outperformed other machine-learning methods that focus only on the target disease.
Meta-Spec’s higher level of accuracy can also be attributed to its ability to rank information. By determining how much a given microbiome or phenotypical characteristic contributes to developing a certain disease and assigning a value to it, the model can make important associations.
For example, age was determined to be the most important factor in detecting cardiovascular disease, where older people are more susceptible. Interestingly, through this ranking feature, the model also linked artificial sweeteners, seafood consumption, and constipation to cardiovascular disease.
Despite its merits, the model also has limitations. “To maintain a high detection performance, Meta-Spec needs a large volume of microbiome data and host metadata. This is a common challenge for deep learning-based approaches,” Su stressed.
To help offset potential data scarcity, Meta-Spec’s developers created a hybrid model that merged data from US- and UK-based cohorts. They found that this cross-cohort approach improved the performance of the model that was trained on local data alone. Since a person’s gut microbiome depends on where they live in the world, a multicohort model could help bridge the geographical gap.
According to Meta-Spec’s developers, once the tool is well-trained by experts, it could become a routine part of a doctor’s office visit in the not-too-distant future.
“We hope Meta-Spec can be applied in hospitals or physical examination centers for early-stage disease prediction,” Su told us. “Meanwhile, Meta-Spec can also help microbiome scientists in the study of host–microbe and microbe–microbe interactions among multiple diseases.”
Eventually, Meta-Spec may even be available as a user-friendly app. “Users could upload the query microbiome data from any computer, and results can also be displayed in a very easy-to-understand way –– just like ChatGPT,” said Su.
Reference:
Wu, et al., Host Variable-Embedding Augment Microbiome-Based Simultaneous Detection of Multiple Disease by Deep Learning, Advanced Intelligent Systems (2023). DOI: 10.1002/aisy.202300342
Feature image credit: Shubham Dhage on Unsplash
Artificial intelligence in disease diagnosis: a systematic literature review, synthesizing framework and future research agenda
Yogesh Kumar,1 Apeksha Koul,2 Ruchi Singla,3 and Muhammad Fazal Ijaz 4
Artificial intelligence can assist providers in a variety of patient care and intelligent health systems. Artificial intelligence techniques ranging from machine learning to deep learning are prevalent in healthcare for disease diagnosis, drug discovery, and patient risk identification. Numerous medical data sources are required to perfectly diagnose diseases using artificial intelligence techniques, such as ultrasound, magnetic resonance imaging, mammography, genomics, computed tomography scan, etc. Furthermore, artificial intelligence primarily enhanced the infirmary experience and sped up preparing patients to continue their rehabilitation at home. This article covers the comprehensive survey based on artificial intelligence techniques to diagnose numerous diseases such as Alzheimer, cancer, diabetes, chronic heart disease, tuberculosis, stroke and cerebrovascular, hypertension, skin, and liver disease. We conducted an extensive survey including the used medical imaging dataset and their feature extraction and classification process for predictions. Preferred reporting items for systematic reviews and Meta-Analysis guidelines are used to select the articles published up to October 2020 on the Web of Science, Scopus, Google Scholar, PubMed, Excerpta Medical Database, and Psychology Information for early prediction of distinct kinds of diseases using artificial intelligence-based techniques. Based on the study of different articles on disease diagnosis, the results are also compared using various quality parameters such as prediction rate, accuracy, sensitivity, specificity, the area under curve precision, recall, and F1-score.
Keywords:
Artificial intelligence, Alzheimer, Cancer disease, Chronic disease, Heart disease, Tuberculosis
Reference:
https://www.ncbi.nlm.nih.gov/pmc/articles/PMC8754556/