Edge AI for Early Detection of Chronic Diseases and the Spread of Infectious Diseases: Opportunities, Challenges, and Future Directions
by Elarbi Badidi
Edge AI, an interdisciplinary technology that enables distributed intelligence with edge devices, is quickly becoming a critical component in early health prediction. Edge AI encompasses data analytics and artificial intelligence (AI) using machine learning, deep learning, and federated learning models deployed and executed at the edge of the network, far from centralized data centers. AI enables the careful analysis of large datasets derived from multiple sources, including electronic health records, wearable devices, and demographic information, making it possible to identify intricate patterns and predict a person’s future health. Federated learning, a novel approach in AI, further enhances this prediction by enabling collaborative training of AI models on distributed edge devices while maintaining privacy. Using edge computing, data can be processed and analyzed locally, reducing latency and enabling instant decision making. This article reviews the role of Edge AI in early health prediction and highlights its potential to improve public health. Topics covered include the use of AI algorithms for early detection of chronic diseases such as diabetes and cancer and the use of edge computing in wearable devices to detect the spread of infectious diseases. In addition to discussing the challenges and limitations of Edge AI in early health prediction, this article emphasizes future research directions to address these concerns and the integration with existing healthcare systems and explore the full potential of these technologies in improving public health.
Reference:
How AI Can Help Diagnose Rare Diseases
New model acts as search engine for large databases of pathology images, has potential to identify rare diseases and therapies
DON NGUYEN
Rare diseases are often difficult to diagnose, and predicting the best course of treatment can be challenging for clinicians. To help address these challenges, investigators from the Mahmood Lab at Harvard Medical School and Brigham and Women’s Hospital have developed a deep- learning algorithm that can teach itself to learn features that can then be used to find similar cases in large pathology image repositories.
Known as SISH (self-supervised image search for histology), the new tool acts like a search engine for pathology images and has many potential applications, including identifying rare diseases and helping clinicians determine which patients are likely to respond to similar therapies. A paper describing the self-teaching algorithm is published in Nature Biomedical Engineering on Oct. 10.
“We show that our system can assist with the diagnosis of rare diseases and find cases with similar morphologic patterns without the need for manual annotations and large datasets for supervised training,” said senior author Faisal Mahmood, assistant professor of pathology at HMS at Brigham and Women’s. “This system has the potential to improve pathology training, disease subtyping, tumor identification, and rare morphology identification.”
Lorem ipsum dolor sit amet, consectetur adipiscing elit. Ut elit tellus, luctus nec ullamcorper mattis, pulvinar dapibus leo.
Science & Medicine, Delivered
Modern electronic databases can store vast reams of digital records and reference images, particularly in pathology, using whole slide images (WSIs). However, the gigapixel size of each individual WSI and the ever-increasing number of images in large repositories means that search and retrieval of WSIs can be slow and complicated. As a result, scalability remains a pertinent roadblock for efficient use.
To solve this issue, the research team developed SISH, which teaches itself to learn feature representations that can be used to find cases with analogous features in pathology at a constant speed regardless of the size of the database.
In their study, the researchers tested the speed and ability of SISH to retrieve interpretable disease subtype information for common and rare cancers. The algorithm successfully retrieved images with speed and accuracy from a database of tens of thousands of WSIs from over 22,000 patient cases, with over 50 different disease types and over a dozen anatomical sites. The speed of retrieval outperformed other methods in many scenarios, including disease subtype retrieval, particularly as the image database size scaled into the thousands of images. Even while the repositories expanded in size, SISH was still able to maintain a constant search speed.
The algorithm, however, has some limitations, including a large memory requirement, limited context awareness within large tissue slides and the fact that it is limited to a single imaging modality.
Overall, the algorithm demonstrated the ability to efficiently retrieve images independent of repository size and in diverse datasets. It also demonstrated proficiency in diagnosis of rare disease types and the ability to serve as a search engine to recognize certain regions of images that may be relevant for diagnosis. This work may greatly inform future approaches to disease diagnosis, prognosis, and analysis.
“As the sizes of image databases continue to grow, we hope that SISH will be useful in making identification of diseases easier,” said Mahmood. “We believe one important future direction in this area is multimodal case retrieval, which involves jointly using pathology, radiology, and genomic and electronic medical record data to find similar patient cases.”
This work was supported in part by National Institute of General Medical Sciences R35GM138216 (to F.M.), Brigham President’s Fund, BWH and MGH Pathology, BWH Precision Medicine Program, Google Cloud Research Grant, and Nvidia GPU Grant Program. Additional support by the Tau Beta Pi Fellowship and the National Cancer Institute Ruth L. Kirschstein National Service Award T32CA251062.
Reference:
https://hms.harvard.edu/news/how-ai-can-help-diagnose-rare-diseases
Artificial intelligence can predict the development of a leading cause of blindness
Artificial intelligence (AI) predicted the development of a leading cause of blindness in new research. A collaboration between Moorfields Eye Hospital in London and Google’s DeepMind and Google Health found that AI predicted the development of wet age-related macular degeneration (wet-AMD) more accurately than clinicians.
Wet-AMD can lead to rapid and severe loss of sight. If detected early enough, treatment can prevent the condition from getting worse. But once damage has occurred, it cannot be reversed.
People attending appointments for wet-AMD in one eye routinely have scans taken of both eyes. In the study, researchers developed a model to predict whether or not wet-AMD would develop in the second eye within six months.
Doctors and optometrists in the study based their predictions on the scans, along with other patient information. Predictions made by the AI model, based on the scans alone, were more accurate.
AI could help doctors diagnose and start treatment earlier. This is expected to give better outcomes for patients.
What’s the issue?
Wet age-related macular degeneration is the leading cause of blindness in the Western world. It tends to affect older people and is most common in the over 50s. It is a degenerative condition and gets worse with time. Left untreated, it leads to loss of central vision, which makes many activities difficult, including reading, recognising faces and driving. The disability caused by this condition can undermine people’s independence.
New, effective treatments have been recommended by the National Institute for Health and Care Excellence (NICE) for use in the NHS in the past decade. But up to half of those diagnosed with late-stage wet-AMD in one eye will go on to develop it in the other eye. Knowing this is likely causes patients a great deal of distress as they are aware of how much it will impact their well-being and independence.
Development of the condition can be rapid and treatment success is dependent on early diagnosis and intervention. But it is not currently possible to predict if or when wet-AMD will affect the second eye.
Analysing scans is time consuming, and this can contribute to delays in diagnosis and treatment.
What’s new?
Researchers at Moorfields Eye Hospital developed an AI model to predict the development of wet-AMD. It analyses digital eye scans (called optical coherence tomography or OCT) used in clinical practice. These scans look at the different layers of eye tissue.
This study included eye scans from 2,795 patients who had wet-AMD in one eye. They were being treated at seven different sites across London between 2012 and 2017.
More than half (60%) of the scans were used to train the AI model. The model was checked in a further 20% scans. This study used the remaining scans to compare the performance of the AI model with that of experts. The model and the experts were asked to predict whether patients would develop wet-AMD in their second eye, within six months of the scan.
The AI model correctly predicted the development of wet-AMD in two in five (41%) patients. It over-predicted wet-AMD in some, who were falsely told they would develop the condition in the second eye. But overall, the model out-performed five out of six experts.
Why is this important?
This is the first example of AI being used to group patients according to their risk of developing a condition (risk stratification). The study found that an AI model was more accurate than doctors and opticians attempting the same task, even though they had access to more information about the patients.
Risk stratification is important for helping hospitals to direct resources towards the patients who need them most. For patients, early intervention for wet-AMD can minimise sight loss, which reduces the impact it will have on their lives, and ultimately on society as a whole.
What’s next?
A clinical trial is planned for 2021. People who already have the condition in one eye will be followed to see how well this model predicts the development of wet-AMD in practice.
You may be interested to read
The full paper: Yim J, and others. Predicting conversion to wet age-related macular degeneration using deep learning. Nature Medicine. 2020;26:892–899
DeepMind published the following blog post to provide some background information to the paper in May 2020: Yim J, and others. Using AI to predict retinal disease progression. DeepMind Blog Post. 18 May 2020
The first paper published from the partnership between researchers at Moorfields Hospital and DeepMind demonstrated that a deep learning model could be used to analyse OCT scans for a variety of eye diseases that affect the retina: De Fauw J, and others. Clinically applicable deep learning for diagnosis and referral in retinal disease. Nature Medicine. 2018;24:1342–1350
DeepMind published the following blog post to announce the 2018 findings: Suleyman M. A major milestone for the treatment of eye disease. DeepMind Blog Post. 13 August 2018
Funding: Pearse Keane is supported by an NIHR Clinician Scientist Award.
Conflicts of Interest: Study authors Pearse Keane and Geraint Rees are paid contractors of DeepMind. Other authors have received fees and funding from various pharmaceutical companies.
Disclaimer: Summaries on NIHR Evidence are not a substitute for professional medical advice. They provide information about research which is funded or supported by the NIHR. Please note that the views expressed are those of the author(s) and reviewer(s) and not necessarily those of the NHS, the NIHR or the Department of Health and Social Care.
How artificial intelligence is making it easier to diagnose disease
Artificial intelligence (AI) can speed up and sometimes outperform clinicians in a variety of tasks and is beginning to be integrated into day-to-day patient care
The use of artificial intelligence (AI) is growing across medicine, particularly in the diagnosis of diseases. Traditional diagnostic approaches are manual and time consuming, for example, reading patient scans is a slow, skill-intensive process and there is a growing shortage of radiologists in the UK to do this work. Using AI can aid clinical decisions and support clinicians by enabling more consistent care delivery across different healthcare settings and freeing up time for other work.
In the same way that doctors learn through education, assignments and experience, AI algorithms – processes or sets of rules for problem-solving operations – must learn their jobs, by being fed data or scans and learning to recognise patterns, analyse data such as medical images and make decisions.
The National Institute for Health and Care Research (NIHR) supports AI innovation and technology from concept to NHS adoption and rollout, through prototype development and real-world testing in health and social care settings.
Predicting drug success for cancer patients
Researchers at The Institute of Cancer Research in London, funded by the NIHR, have created a prototype test that can predict which drug combinations are likely to work for cancer patients in as little as 24-48 hours. They use AI to analyse large-scale data from tumour samples and can predict patients’ response to drugs more accurately than current methods.
Analysing the genetic makeup of tumours can reveal the mutations that encourage growth, and some of these can be targeted with treatment. This alone is not enough to select drug combinations, and the new test also examines molecular changes in the tumour, and how they interact with each other in response to treatments.
The AI works in two stages, first by predicting how cells are to individual cancer drugs, looking in particular at three genetic markers, and then predicting how they will respond to combinations of two drugs.
It can process information quickly, turning around results in just two days, and has the potential to guide doctors on which treatments are most likely to benefit individual cancer patients.
Reducing COVID-19 spread in hospitals
At the University of Oxford, a tool has been developed, that can rule out COVID-19 infection within an hour of people arriving at a hospital. It is far quicker than the 24 hours required for a PCR test, and more reliable than lateral flow tests. Better yet, it uses only the data that is already collected within a patient’s first hour in hospital.
When people arrive in hospital their body temperature, blood pressure and heart rate are measured and blood is taken. The AI tool was trained using this information from 115,000 patients along with their PCR test results to say whether they had COVID-19 or not.
Its performance was then evaluated by estimating the COVID-19 status of all patients arriving at two hospitals’ over a two week period. Of over 3,300 patients arriving in the emergency department and 1,715 patients who were admitted, the AI agreed with the PCR test nine out of ten times, and was 98% accurate at ruling out COVID-19.
The software could be used to reduce the spread of the virus in hospitals and speed up treatment for people who are not infected with the virus. It is being tested in more hospital settings to evaluate how well it works in real world hospital conditions. This research was supported by the NIHR.
Continually improving care
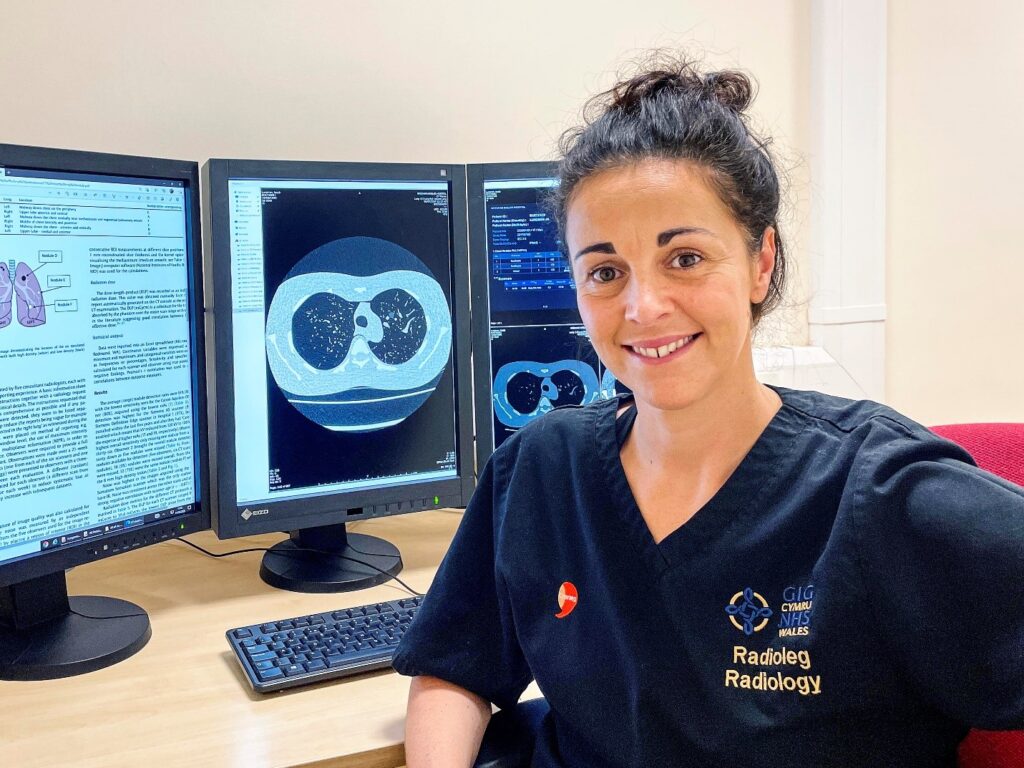
Dr Jenna Tugwell-Allsup is a research radiographer at Betsi Cadwaladr University Health Board in North Wales, who has long championed research as a way to help patients. Her past work revealed that giving patients a video clip to watch with information explaining what an MRI scan involves, what noise to expect, and the importance of staying still. She found that this video was more effective in reducing patients’ anxiety than providing written information.
Her current research focus is on AI including the MIDI trial, which is investigating whether an AI tool can identify abnormalities on MRI head scans. The AI tool is being developed and tested on patient head scans as well as healthy volunteers’ scans to teach it to identify abnormalities more quickly so that these can be prioritised for assessment by a clinician. She has also received funding to conduct a study to determine if an AI algorithm can reduce time to diagnosis of lung cancer by retrospectively assessing the chest x-rays of known lung cancer patients.
Jenna has set up a local AI Working Group within Radiology and will be collaborating with other similar groups to streamline the process of working with AIs. She says: “Research pushes the barriers of what we think we know. Just because we are doing something well, doesn’t mean we cannot do it even better. I hope my research has guided and influenced others in radiography to think critically about the way we work and how we can continually improve care.”
AI studies
Because AI technologies can analyse datasets and scans in seconds, there is great value in training them to read patient scans and assist in diagnosis and predicting the best treatments. The NIHR is currently funding trials to see if algorithms can support pathologists in reading images of prostate cancer biopsies, help to reliably identify coronary artery disease by reading the imaging used to diagnose it, and to correctly recognise ‘glue ear’, a common childhood ear infection where the empty middle part of the ear canal fills up with fluid. This condition is also known as otitis media with effusion, and can cause hearing impairment and disability.
The NIHR ensures that patients and clinicians are actively involved in the development of these new tools so that they can be implemented in practical settings across the NHS. This also ensures that they are designed to mitigate the potential for bias in these algorithms – both in terms of the data they are trained on but also any incorrect assumptions as to how accessible they will be by less privileged groups.
How you can get involved with research
Advancements in AI to speed up diagnoses is just one of many areas of research taking place across the UK. If you want to know more or are keen to can get involved with research, take a look at the studies we are running on our Be Part of Research website. There you can search our list to find a study that sounds right for you.
If you want more information on what to expect, you can find out more about what happens in a study.
There are other ways to get involved in research if taking part in a study doesn’t feel right for you at the moment.
Reference:
https://bepartofresearch.nihr.ac.uk/articles/artificial-intelligence/
Evaluation of artificial intelligence techniques in disease diagnosis and prediction
A broad range of medical diagnoses is based on analyzing disease images obtained through high-tech digital devices. The application of artificial intelligence (AI) in the assessment of medical images has led to accurate evaluations being performed automatically, which in turn has reduced the workload of physicians, decreased errors and times in diagnosis, and improved performance in the prediction and detection of various diseases. AI techniques based on medical image processing are an essential area of research that uses advanced computer algorithms for prediction, diagnosis, and treatment planning, leading to a remarkable impact on decision-making procedures. Machine Learning (ML) and Deep Learning (DL) as advanced AI techniques are two main subfields applied in the healthcare system to diagnose diseases, discover medication, and identify patient risk factors. The advancement of electronic medical records and big data technologies in recent years has accompanied the success of ML and DL algorithms. ML includes neural networks and fuzzy logic algorithms with various applications in automating forecasting and diagnosis processes. DL algorithm is an ML technique that does not rely on expert feature extraction, unlike classical neural network algorithms. DL algorithms with high-performance calculations give promising results in medical image analysis, such as fusion, segmentation, recording, and classification. Support Vector Machine (SVM) as an ML method and Convolutional Neural Network (CNN) as a DL method is usually the most widely used techniques for analyzing and diagnosing diseases. This review study aims to cover recent AI techniques in diagnosing and predicting numerous diseases such as cancers, heart, lung, skin, genetic, and neural disorders, which perform more precisely compared to specialists without human error. Also, AI’s existing challenges and limitations in the medical area are discussed and highlighted.
Keywords:
Artificial intelligence, Deep learning, Machine learning, Diseases diagnosis, Medical image processing
Reference:
https://www.ncbi.nlm.nih.gov/pmc/articles/PMC9885935/